My Kaggle Elite 8 probs for Men and Women
Lost a few spots yesterday. Currently in 75th. I still need UConn and South Carolina to win in all in the Men’s and Women’s tournament, respectively.

Pre tournament probabilities that the remaining men’s teams would reach the Final 4:
UConn: 56.0% (100% in my aggressive Bracket)
Purdue: 51.8%
Tennessee: 18.2%
Alabama: 10.5%
Illinois: 8.11% (0% in aggressive Bracket)
Duke: 6.23%
Clemson: 2.87%
NC State: 0.87%
Pre tournament probabilities that the remaining men’s teams would reach the Final 4:
South Carolina: 82.5%% (100% in my aggressive Bracket)
Oregon St: 8.09% (0% in my aggressive Bracket)
Texas: 28.0%
NC State: 13.8%
LSU: 18.0%
Iowa: 28.4%
UConn: 27.5% / Duke: 1.37%
USC: 33.4% /Baylor: 4.21%
Cheers.
Kaggle March Madness Update – March 26, 2024
I thought I finally understood the scoring for this contest, but I can’t reproduce my score on the leaderboard. So I have no idea. But I’m at 141 after the first weekend. Unlikely to cash, looking to just finish top 100.

Sweet Sixteen probabilities for women: (Men’s Sweet Sixteen probabilities are here):
South Carolina: 91.9% (100% in aggressive bracket)
Indiana: 5.83% (0% in aggressive bracket)
Oregon St: 50.1%
Notre Dame: 41.9%
Gonzaga: 21.3%
Texas: 56.8%
NC State: 27.9%
Stanford: 65.4%
USC: 60.1%
Baylor: 12.2%
Duke: 5.1%
UConn: 47.2%
Colorado: 17.1%
Iowa: 56.6%
LSU: 34.0%
UCLA: 57.2%
Cheers.
Kaggle March Madness Update
Well, I’ve gone up in the rankings every scoring update. From 233rd to 170th to currently in 126th. Just about top 15%. That’s positive. And with the new scoring system, there is a ton of room to move up (and DOWN) very quickly.



Probabilities for women’s games today (remember these are pre-tournament probabilities that these teams would reach the Sweet Sixteen, NOT single game win probabilities):
Notre Dame: 79.33%
Ole Miss: 17.45%
NC State: 78.33%
Tennessee: 20.37%
Syracuse: 18.67%
UConn: 76.76%
Oklahoma: 26.11%
Indiana: 69.29%
West Virginia: 11.69%
Iowa: 83.15%
Creighton: 9.48%
UCLA: 85.15%
Kansas: 9.57%
USC: 85.8%
Utah: 40.58%
Gonzaga: 55.04%
And here are my probabilities for the men’s games next Thursday and Friday:
Thursday
Clemson: 7.75%
Arizona: 26.47%
San Diego St: 6.04% (0% in my aggressive bracket)
UConn: 71.53% (100% in my aggressive bracket)
Alabama: 21.37%
North Carolina: 51.43%
Illinois: 27.09%
Iowa St: 46.87%
Friday
NC State: 4.52%
Marquette: 38.57%
Gonzaga: 6.86%
Purdue: 69.26%
Duke: 12.87%
Houston: 69.82%
Creighton: 21.28%
Tennessee: 44.22%
In my aggressive bracket I have South Carolina and UConn winning the men’s and women’s titles with probability 100%. If that happens I pick up 0 loss for all those games in all 6 rounds. And 0 loss in the finals would be absolutely massive in reducing my score as it would be a full 1/6th of my score with 0 loss. So let’s go UConn and South Carolina!
Cheers.
March 24 Kaggle Probabilities
Currently in 170th. Better than yesterday.

My Kaggle probabilities for the men’s games today:
(Remember, these aren’t probabilities that these teams will win this particular game, it’s the pre-tournament probability that they would make it to a particular round.)
Colorado: 18.3%
Marquette: 60.27%
Purdue: 84.1%
Utah St: 7.62%
James Madison: 11.67%
Duke: 50.77%
Clemson: 17.55%
Baylor: 53.01%
Grand Canyon: 13.95%
Alabama: 49.01%
Northwestern: 4.51% (0% in my aggressive bracket)
UConn: 88.83% (100% in aggressive bracket)
Texas A&M: 6.36%
Houston: 83.74%
Yale: 3.25%
San Diego St: 31.47%
My Kaggle probabilities for the women’s games today:
(Remember, these aren’t probabilities that these teams will win this particular game, it’s the pre-tournament probability that they would make it to a particular round.)
Colorado: 42.38%
Kansas St: 53.03%
South Carolina: 97.7% (100% in aggressive bracket)
North Carolina: 0.79% (0% in my aggressive bracket)
Ohio St: 77.5%
Duke: 19.72%
MTSU: 2.73%
LSU: 78.24%
Nebraska: 10.95%
Oregon St: 83.56%
Alabama: 10.63%
Texas: 82.88%
Iowa St: 6.96%
Stanford: 89.52%
Baylor: 36.57%
Virginia Tech: 57.18%
Go UConn.
Cheers.
NCAA Tournament Stuff – March 23,2024
The real stories everyone should be talking about are how David Carr beat Keegan O’Toole in the semi-finals of the NCAA wrestling tournament at 165, Carter Starocci had to wrestle former national champions back to back in the quarter- (Mekhi Lewis) and semi-finals (Shane Griffith) and he beat them both, and Drake Ayala of Iowa made the finals keep Iowa’s streak of THIRTY THREE years in a row with a national finalist alive.
But you probably want to read about basketball, huh?
So here’s a fun, simple data viz of wins by seed:
Below is a plot of wins by seed in the first round of the men’s NCAA tournament. The 6 and 8 seeds combined for only two total wins ((6)Clemson and (8)Utah State). At least one of every seed 1 through 14 advanced and seed 9 through 12 went went a combined 9-7. In total the lower seed won 11 of the first 32 games.

As for the Kaggle contest, I’m currently in 233 out of 820 (top 30%). Not great, but also not terrible either. There should be a lot more shuffling of the leaderboards in these later rounds with the changes in scoring, so it’s going to be way more exciting than past contests, I think. At least in terms of chaos.

Here are my probabilities for the women’s games today:
Tennessee: 78.2%
UConn: 100%
Kansas: 55.6%
Indiana: 98.0%
Notre Dame: 99.1%
NC State: 98.4%
Iowa: 100%
Syracuse: 72.0%
Oklahoma: 76.0%
USC: 99.3%
Ole Miss: 72.7%
West Virginia: 63.0%
Creighton: 56.2%
Gonzaga: 96.9%
UCLA: 99.0%
Utah: 76.0%
And here are my Kaggle probabilities for the men’s games today:
(Remember, these aren’t probabilities that these teams will win this particular game, it’s the pre-tournament probability that they would make it to a particular round.)
Arizona: 51.6%
Dayton: 19.5%
Kansas: 47.4%
Gonzaga: 35.1%
UNC: 73.9%
Michigan St: 13.3%
NC State: 14.0%
Oakland: 1.78%
Iowa St: 71.6%
Washington St: 11.9%
Tennessee: 69.5%
Texas: 17.7%
Illinois: 55.5%
Duquesne: 5.83%
Creighton: 47.5%
Oregon: 16.8%
Finally, and most importantly, my numbers (8 and 2) hit in the Utah State-TCU game in NCAA sqaures. So worst case scenario, I only lose half my square buy-in. Lettttttt’s gooooooooooooo.
Cheers.
My completely uninformed guide to March Madness and some thoughts on my kaggle entry
I submitted my March Madness Machine Learning Mania today. My two entries consist of picks made using the actual spread for first round games and then a simple Bradley-Terry model for the games past that. In my second bracket, which I called the “aggressive” one, I picks UConn in the men’s bracket and South Carolina in the women’s bracket to win each round (and thus the tournament) with probability 1. So is UConn and South Carolina win, maybe I have a shot at winning. I also manually adjusted two teams on the women’s side (South Carolina and USC). The problem with South Carolina is that they haven’t lost any games and Bradley-Terry basically can’t handle that. I adjusted their regression coefficients to match the market price that they win the tournament. I also adjusted USC on the women’s side because they were way off their market price too. I didn’t make any adjustments to the men’s side because the futures prices were generally in the ball park with what I was estimating (i.e. there are three truly top teams in the men’s bracket (Purdue, UConn, Houston), then a sizable gap down to the next team (which I think is Iowa State. North Carolina got a gift of a 1 seed.)
I’m really excited about the new scoring system for Kaggle this year. And I think they got the scoring system right. A few weeks ago I believe I read that the scoring system was going to be average bracket score with traditional bracket scoring (1-2-4-8-16-32). My first thought when I saw this was that the best strategy is to just enter one bracket and hope. I think other people figured this out and they change it to a Brier score metric. But what they really got right this year is that that don’t take the average over all the GAMES, they take the average over the 6 ROUNDS. This weights the game in the finals much more heavily than a game in the first round, much closer to traditional bracket games.
Anyway, here are some probabilities below are based on my 10000 brackets that I submitted:
To win the championship:
UConn – 29.3%
Houston – 20.9%
Purdue – 19.4%
Iowa St – 5.19%
North Carolina – 4.96%
Tennessee – 3.39%
Marquette – 2.49%
Auburn – 1.94%
Illinois – 1.51%
Baylor – 1.43%
Everyone else < 1%
To make the finals:
UConn – 45.5%
Houston – 34.8%
Purdue – 32.4%
North Carolina – 12.6%
Iowa St – 11.4%
Tennessee – 7..92%
Marquette – 6.51%
Auburn – 5.8%
Illinois – 4.54%
Baylor – 4.34%
Arizona – 3.16%
Alabama – 2.68%
South Carolina – 2.46%
Kansas – 2.31%
Kentucky – 2.13%
Creighton 2.03%
Everyone else < 2%
And finally, here are my pre-tournament rankings 1 through UMass:
Rank | TeamName |
1 | Connecticut |
2 | Houston |
3 | Purdue |
4 | Iowa St |
5 | North Carolina |
6 | Tennessee |
7 | Auburn |
8 | Marquette |
9 | Illinois |
10 | South Carolina |
11 | Baylor |
12 | Kansas |
13 | Utah St |
14 | Creighton |
15 | Arizona |
16 | Duke |
17 | Nevada |
18 | Kentucky |
19 | Alabama |
20 | San Diego St |
21 | BYU |
22 | Florida |
23 | Texas Tech |
24 | New Mexico |
25 | Wisconsin |
26 | Gonzaga |
27 | Nebraska |
28 | Colorado St |
29 | Dayton |
30 | Clemson |
31 | Virginia |
32 | Mississippi St |
33 | Boise St |
34 | Texas |
35 | St Mary’s CA |
36 | TCU |
37 | Drake |
38 | Oklahoma |
39 | Grand Canyon |
40 | Northwestern |
41 | Colorado |
42 | Texas A&M |
43 | Washington St |
44 | Indiana St |
45 | Pittsburgh |
46 | NC State |
47 | FL Atlantic |
48 | Syracuse |
49 | Providence |
50 | Michigan St |
51 | Oregon |
52 | St John’s |
53 | James Madison |
54 | Seton Hall |
55 | Mississippi |
56 | Kansas St |
57 | Ohio St |
58 | Indiana |
59 | Princeton |
60 | Wake Forest |
61 | Cincinnati |
62 | Iowa |
63 | Butler |
64 | Virginia Tech |
65 | Villanova |
66 | Samford |
67 | Richmond |
68 | Duquesne |
69 | McNeese St |
70 | Utah |
71 | Memphis |
72 | Loyola-Chicago |
73 | South Florida |
74 | UNLV |
75 | Florida St |
76 | Boston College |
77 | Xavier |
78 | UCF |
79 | LSU |
80 | Georgia |
81 | VCU |
82 | Minnesota |
83 | UAB |
84 | Washington |
85 | Bradley |
86 | San Francisco |
87 | Appalachian St |
88 | Arkansas |
89 | Rutgers |
90 | Cornell |
91 | Vermont |
92 | Miami FL |
93 | Col Charleston |
94 | Maryland |
95 | Penn St |
96 | Georgia Tech |
97 | St Joseph’s PA |
98 | Yale |
99 | USC |
100 | UC Irvine |
101 | St Bonaventure |
102 | Santa Clara |
103 | George Mason |
104 | Massachusetts |
NCAA basketball squares
We all know about Super Bowl squares. But last night my friend asked me to be in an NCAA squares pool. I asked him how it worked and he explained to me that it’s the same as a Super Bowl pool, but you have the same numbers for all 63 games (not the stupid F-ing play-in games). So naturally, I wanted to know what the best numbers were. Here are the results based n data from 1985 – 2021, which is just the data that I happened find on my computer first.
The worst set of numbers are the ones that are the same. Since it’s based on only final score, the only way these numbers will hit if a team wins by exactly 10, 20, 30, etc. Also, note that in the pct column, if all squares were equally likely, the pct should be exactly 1% for every square. So even in the worst case (i.e. 1-1), the percentage isn’t that far off from what you would expect if each square was equally likely.

The best numbers are combinations that are all 2 or 3 digits away with 1-8, 0-8, 3-1, 5-2 and 9-6 being the top five all with probabilities that are essentially the same.
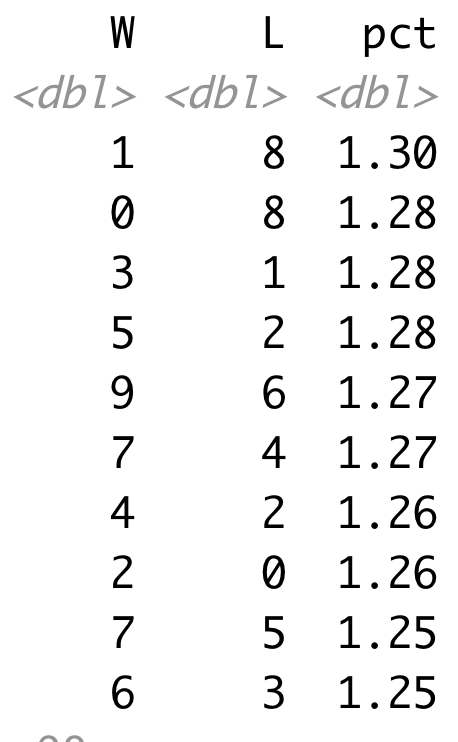
To better see the pattern, I made a 2d histogram for all the scores. Here, red, white, and green indicate combinations that are worse, about the same, and better than 1%. You can see the dark green band running up the diagonal of combinations that are 2 and 3 apart.
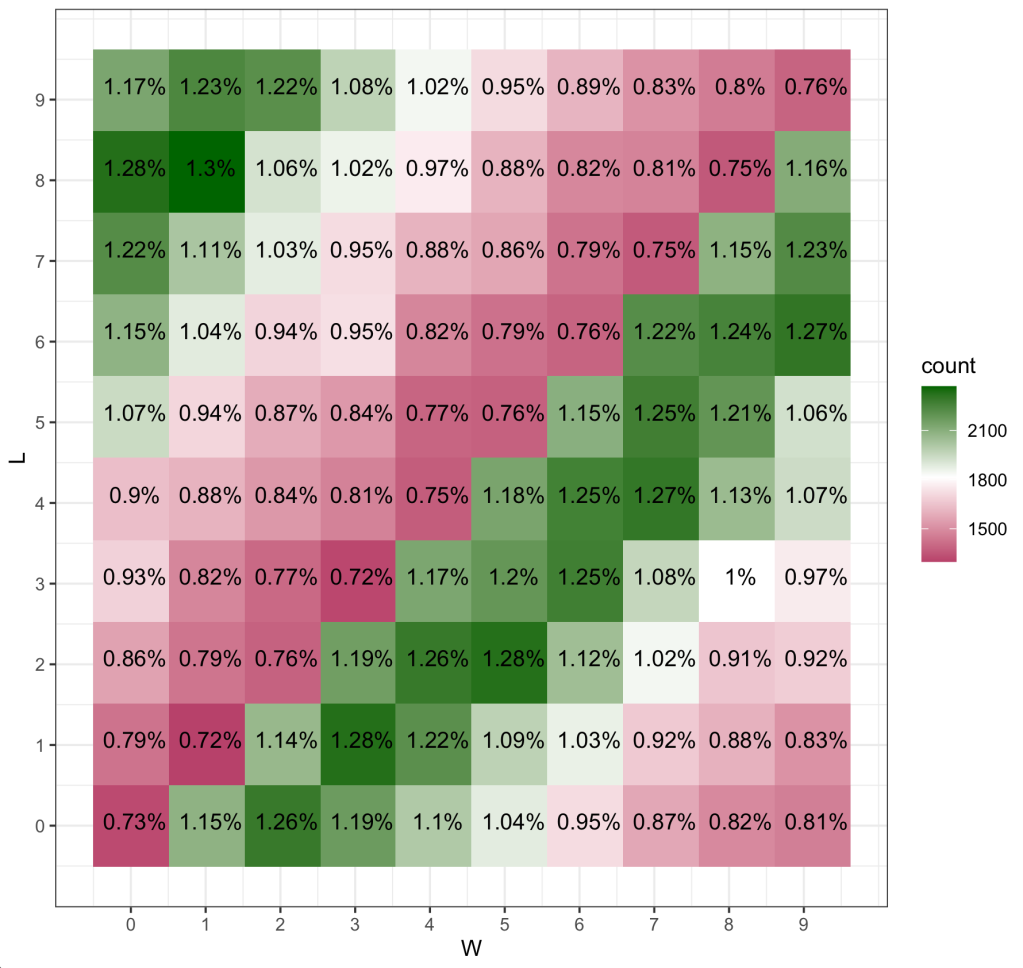
And just for comparison sake, here is the same plot with NFL scores:

Happy gambling in March!
Cheers.
STRAIN and Editors Choice Collection
My article “Here Comes the STRAIN: Analyzing Defensive Pass Rush in American Football with Player Tracking Data” with Quang Nguyen and Ron Yurko was selected for inclusion in the AMSTAT News Editor’s Choice Collection. This means you can read the full article for free (Note: You can always read any of my articles for free. If you just contact me, I’ll send you a free copy. Never ever ever pay the publisher fort access to my work, please).
While glancing through the list there were a few articles that I am particulars interested in reading:
- “The Twentieth-Century Reversal: How Did the Republican States Switch to the Democrats and Vice Versa?” by Andrew Gelman. I’m SO SICK of hearing people talk about how the GOP is the “Party of Lincoln”. Yeah, that’s technically true, but that entirely ignores history. I can’t wait to read this article.
- “Integrating Data Science Ethics into an Undergraduate Major: A Case Study” by Benjamin S. Baumer, Randi L. Garcia, Albert Y. Kim, Katherine M. Kinnaird, and Miles Q. Ott. This author list is a who’s who of people I turn to when I am developing my courses. Baumer’s book (with Kaplan and Horton) is the foundation for our undergraduate Introduction to Data Science with R (DSCI101) course and our graduate version Introduction to Data Science (with R and Python) (DSCI401) course.
- “Changes in Crime Rates During the COVID-19 Pandemic” by Mikaela Meyer, Ahmed Hassafy, Gina Lewis, Prasun Shrestha, Amelia M. Haviland.
Cheers.
Covid-19 and myocarditis
“People on the internet” (i’m talking about the anti-vax morons) scream about how myocarditis is a side effect of the vaccine, but big pharma doesn’t want you to know about. Chief, it’s literally listed on the CDC website.

And if you are that worried about myocarditis, you know what causes myocarditis at much higher rates: Actual Covid-19!
Cheers.
The thing about multiple hypothesis testing that has always bothered me
Here is what’s always bothered me about multiple hypothesis testing.
Let’s say there are 10 hypothesis tests and they come back and 3 of them are significant at the 0.05 level. However, after correcting for multiple test using, for simplicity, a Bonferroni correction, none of these tests are significant. (Assume that it doesn’t matter what method you use to correct, but after correction you find nothing significant). So when an individual researcher does these tests together, they have to report that they found nothing significant.
Now let’s say that 10 different researchers do one of these ten tests each, and they get the exact same p-values. Now 3 of these researchers will get “significant” results because they are only doing one test. So three of these researchers publish their results.
It’s the same exact set of tests with the same exact p-values. But if a single researcher does it, there is nothing significant. And if they did report something significant they would be accused of p-hacking (and rightly so). But if 10 different independent researchers each do one of the tests, they will come up with 3 out of the 10 tests as significant. Same data. Same results. Same p-values. Different conclusions based on who performed the test. Weird, right?
Cheers.